The Intractable Downside of AI Hallucinations
[ad_1]
Artificial Intelligence & Machine Learning
,
Next-Generation Technologies & Secure Development
Options to Gen AI’s ‘Inventive’ Errors Not Enterprise-Prepared, Say Specialists
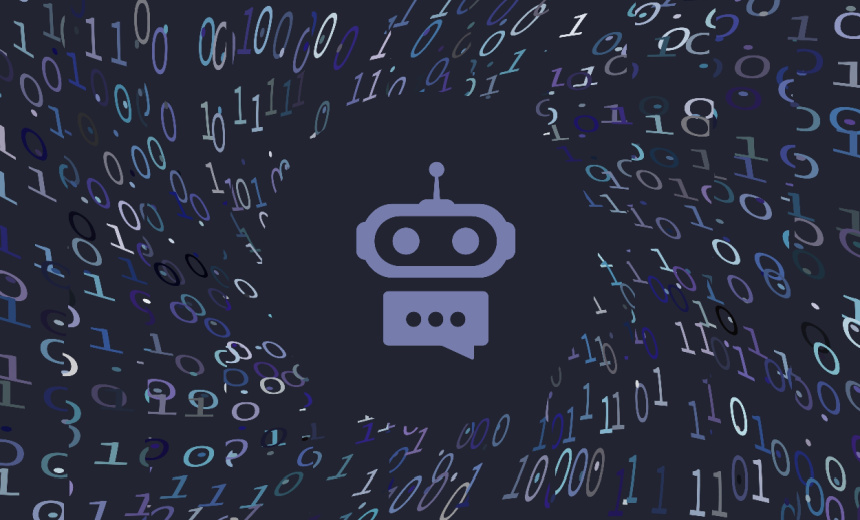
The tech industry is rushing out products to tamp down artificial intelligence models’ propensity to lie faster than you can say “hallucinations.” But many experts caution they haven’t made generative AI ready for scalable, high-precision enterprise use.
See Also: Accelerating defense missions with a global data mesh
Hallucinations arguably are gen AI’s biggest downside – the typically laughably incorrect, typically viral and typically harmful or deceptive responses that enormous language fashions spit out as a result of they do not know higher. “The problem is that these fashions predict phrase sequences with out really understanding the info, making errors unavoidable,” mentioned Stephen Kowski, subject CTO at SlashNext.
Tech firms’ resolution has been to search for methods to cease hallucinations from reaching customers – layering tech on prime of tech. Options reminiscent of Google’s Vertex AI, Microsoft’s correction capability and Voyage AI use assorted approaches to enhance the accuracy of LLM outputs.
The correction functionality goals to curb hallucinations by boosting the output’s reliability by “grounding” the responses to particular sources with reliable info the LLMs can entry, Microsoft informed Data Safety Media Group. “For instance, we floor Copilot’s mannequin with Bing search information to assist ship extra correct and related responses, together with citations that enable customers to lookup and confirm info,” a spokesperson mentioned.
However approaches fall quick if the expectation is for high-precision outcomes, mentioned Ram Bala, affiliate professor of AI and analytics at Santa Clara College.
“Consider it this manner: LLMs are all the time dreaming. Generally these desires are actual. How is this beneficial? It’s extremely helpful once you need inventive output like writing a depart of absence letter, however enterprise purposes don’t all the time want this creativity,” he informed ISMG.
Implementing safeguards for all use instances is usually cost-prohibitive. Many firms want to prioritize velocity and breadth of deployment over accuracy, mentioned Kowski.
Specialists mentioned a layered method to stopping hallucinations can stymie hallucinations for frequent shopper use instances the place an incorrect response may trigger hurt or be inappropriate since builders have sufficient information to, as an illustration, cease fashions from once more advising customers to place glue on pizza to cease cheese from sliding off (see: Breach Roundup: Google AI Blunders Go Viral). “It is one of many causes we do not hear as many complaints about ChatGPT as we did two years in the past,” Bala mentioned.
However the method of layering anti-hallucination options in AI fashions is insufficient for nuanced, enterprise-specific calls for. “Enterprises have many complicated issues to resolve and loads of nuanced guidelines and insurance policies to observe. This requires a deeper customized method that lots of the massive tech firms will not be able to put money into,” he mentioned.
Specialists additionally argue that no developments in know-how can absolutely obliterate hallucinations. It is because hallucinations aren’t bugs within the system however byproducts of how AI fashions are skilled to function, mentioned Nicole Carignan, vp of strategic cyber AI at Darktrace.
Hallucinations happen as a result of gen AI fashions, significantly LLMs, use probabilistic modeling to generate output primarily based on semantic patterns of their coaching information. Not like conventional information retrieval, which pulls verified info from established sources, fashions generate content material by predicting what’s prone to be appropriate primarily based on earlier information. Kowski mentioned some analysis concludes it might be mathematically inconceivable for LLMs to be taught all computable features.
Various Approaches
Whereas massive tech has largely centered on broad-scale, generalized options, a number of startups are taking a extra focused method to deal with hallucinations. Bala described two main methods rising amongst these smaller gamers: permitting enterprises to construct customized guidelines and prompts, and growing domain-specific purposes with curated data bases. Some startups allow firms to encode their very own guidelines inside LLMs, adapting AI to fulfill explicit wants. Different startups deploy area experience to create data graphs which might be paired with retrieval-augmented technology, additional anchoring AI responses in verified info. RAG lets LLMs reference paperwork exterior coaching information sources when responding to queries. Whereas these strategies are nonetheless nascent, Bala mentioned he anticipated speedy developments within the coming 12 months.
Specialists mentioned that supervised machine studying, which is extra structured than the probabilistic method of gen AI, tends to yield extra dependable outcomes for purposes requiring excessive accuracy.
To harness AI’s advantages whereas mitigating hallucinations, Carignan recommends a multi-faceted method. Sturdy information science ideas reminiscent of rigorous testing and verification, mixed with layered machine studying approaches, will help scale back errors. However know-how alone is not sufficient, she mentioned. Safety groups have to be embedded all through the whole course of to make sure AI security and workers are educated about AI’s limitations.
[ad_2]
Source link